By Sursha Wu
What is AI automation?
AI automation is the use of AI to perform tasks, make decisions, and streamline processes without the need for constant human intervention. It marks a shift from traditional automation—which depends on fixed rules and repetitive programming—to intelligent systems that can “learn, adapt, and optimize” over time.
AI automation draws from various technologies such as machine learning, computer vision, and natural language processing. These tools allow systems to process large amounts of data, recognize patterns, understand human input, and make informed decisions in real time.
AI automation tools
AI-Powered Production Scheduling
As market conditions change more rapidly, the need for real-time and automated scheduling continues to grow. AI can analyze factors such as inventory, orders, and production capacity to generate predictive schedules—saving time, reducing manual effort, and potentially lowering costs through more accurate planning.
AI Vision Systems
AI vision plays a critical role in modern manufacturing by enabling machines to visually understand and analyze complex producing environments.
- Smarter Safety Monitoring
AI vision helps enforce safety by automatically checking if workers wear required gear like helmets and gloves. It also sets virtual safety zones around machines, reducing the risk of accidents in human-machine collaboration areas
- More Accurate Defect Detection
Traditional AOI systems often struggle with high false rejection rates. AI vision, on the other hand, can recognize patterns and understand product features more flexibly. This results in better identification of actual defects—improving both accuracy and product quality.
Human actions on the line are often unpredictable and hard to track—but AI vision makes them visible. AI vision tracks whether workers follow each step of the SOP and flags issues like skipping tasks or leaving stations in real-time. This gives managers 100% control over what’s happening on the floor and helps prevent small issues from becoming major production problems.
Learn more about AI vision application in IPQC
Predictive Maintenance Platforms
Unplanned downtime is a serious risk in manufacturing. AI-powered platforms analyze machine data—such as temperature, vibration, and performance trends—to detect early signs of failure and recommend optimal maintenance timing. This minimizes disruptions and reduces maintenance costs.
Digital Twins
By simulating a factory’s physical processes virtually, digital twins help engineers test new processes or layout changes without disrupting live production.
Benefits of AI automation for factory
AI automation helps factories become more efficient and data-driven.
For manufacturers, cost reduction and efficiency improvement are top priorities when investing in new tools. However, the long-term value of AI automation lies not just in quick ROI, but in how deeply it integrates into daily operations and decision-making across the entire organization.
Read the Success Case: Global EMS adopted AI Automation in Factory
Handling More Complex Tasks
AI can handle far more complex tasks compared to traditional automation or single-function systems.
For example, AI can analyze video, acquire process knowledge, and then automatically assist industrial engineering (IE) in diagnosing root causes of issues and generating reports.
This capability not only enables AI and automation to tackle tasks that were previously impossible to automate but also frees up managers and operators to focus on truly challenging and complex problems, thereby improving overall efficiency and quality in management.
Enhancing Decision-Making Power
AI automation empowers better, faster decisions across all levels of management.
With automated data collection and real-time reporting, managers gain greater visibility and control over production activities and operational performance.
For manufacturers operating across Southeast Asia, this is especially valuable. Many headquarters are located in Singapore, Taiwan, or China, while factories are based in countries like Thailand or Vietnam. Without an integrated and automated data system, global high-level management often lack access to what’s actually happening on the ground.
With AI automation, production data becomes instantly accessible—no matter where managers are. This empowers proactive oversight, early disruption detection, and unified management across multiple sites.
How AI is used for automation: 3 cases in SEA
Download the Full Report: 2025 Southeast Asia Manufacturing Dynamic
Southeast Asia has become a strategic region for global manufacturers, not only due to its cost advantages but also its geopolitical relevance, skilled labor availability, and expanding supply chain ecosystems.
However, success in setting up operations goes beyond simply building a factory. Manufacturers must evaluate whether the local environment supports sustainable operations through workforce readiness, mature supply chain networks, and efficient logistics infrastructure.
In response, AI automation is proving essential for helping companies manage complexity, improve operational stability, and scale intelligently in this fast-evolving region.
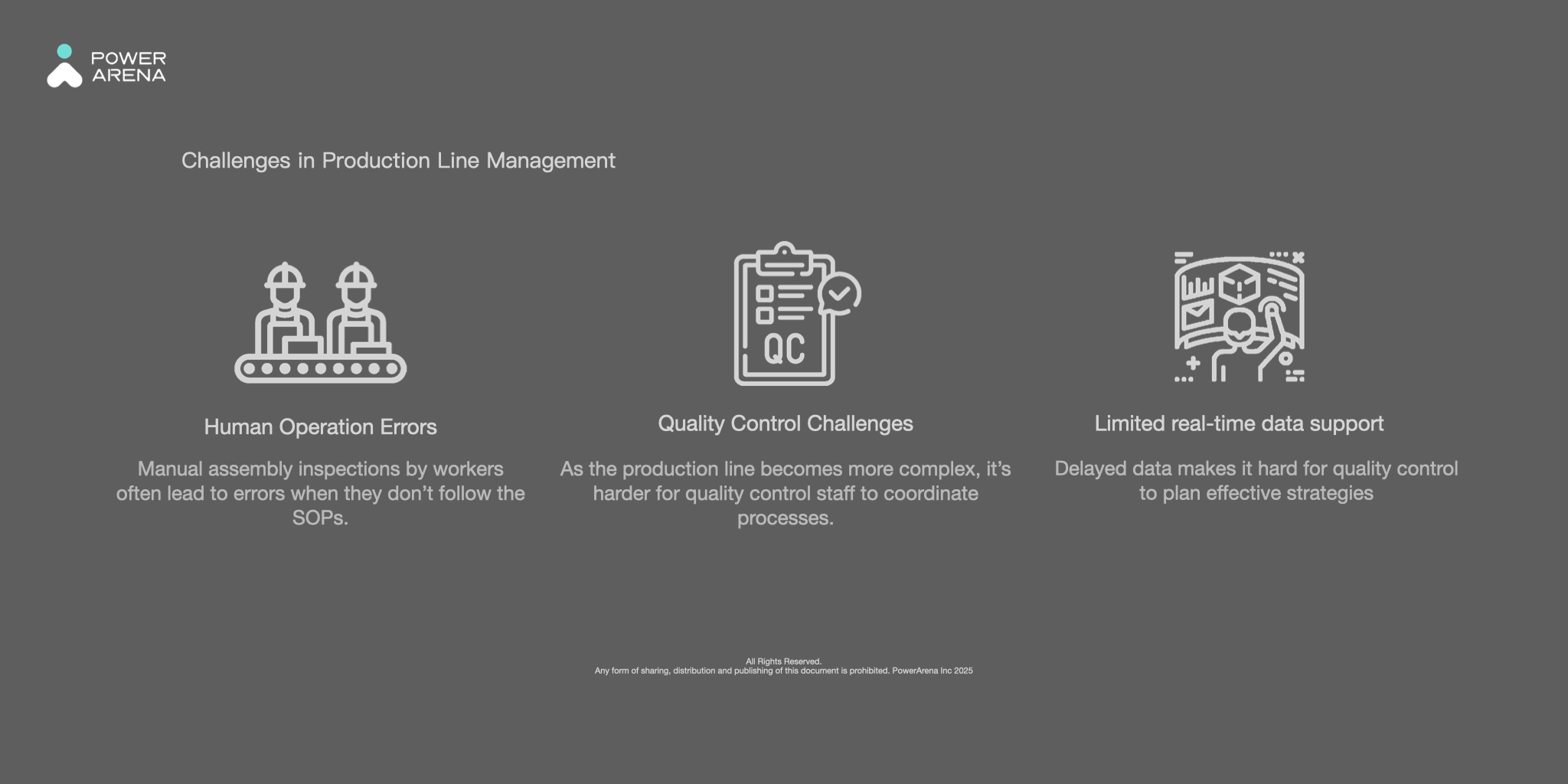
Challenges in Production Line Management
Case 1: Smarter Floors, Faster Fixes
Read the Case Study: AI vision for line management
In northern Vietnam, an electronics manufacturer was facing inconsistent production output due to frequent onboarding of new operators. Traditional tracking systems couldn’t pinpoint where or why performance fluctuations were happening along the line.
To solve this, the factory introduced AI automation through AI vision systems installed at key workstations. Cameras captured real-time production activities, while the system automatically tracked operator behavior and collected key data—such as cycle time, process time, and idle time. This data was then analyzed and transformed into visual reports, helping managers quickly understand what was happening on the floor.
By automating data collection and analysis, the factory made production monitoring simpler and more immediate. Managers could now easily identify abnormal stations and take corrective actions, improving both response time and overall line performance.
Case 2: Cutting Energy Costs with AI
A Thai manufacturer operating energy-intensive equipment across multiple shifts faced rising electricity costs and occasional unplanned downtime. Manual energy audits failed to uncover the root causes of inefficiency.
By implementing an AI-powered energy management system, the factory gained real-time visibility into energy consumption at the equipment level. The AI detected peak usage patterns and identified machinery that could be rescheduled to avoid demand surges.
Case 3: When Quality Can’t Be Seen, AI Vision Steps In
If Poka Yoke is implemented effectively during the manufacturing process, many quality control tasks can even be eliminated.
— Junny Lu, Former Executive Factory Head & PowerArena AI System Consultant
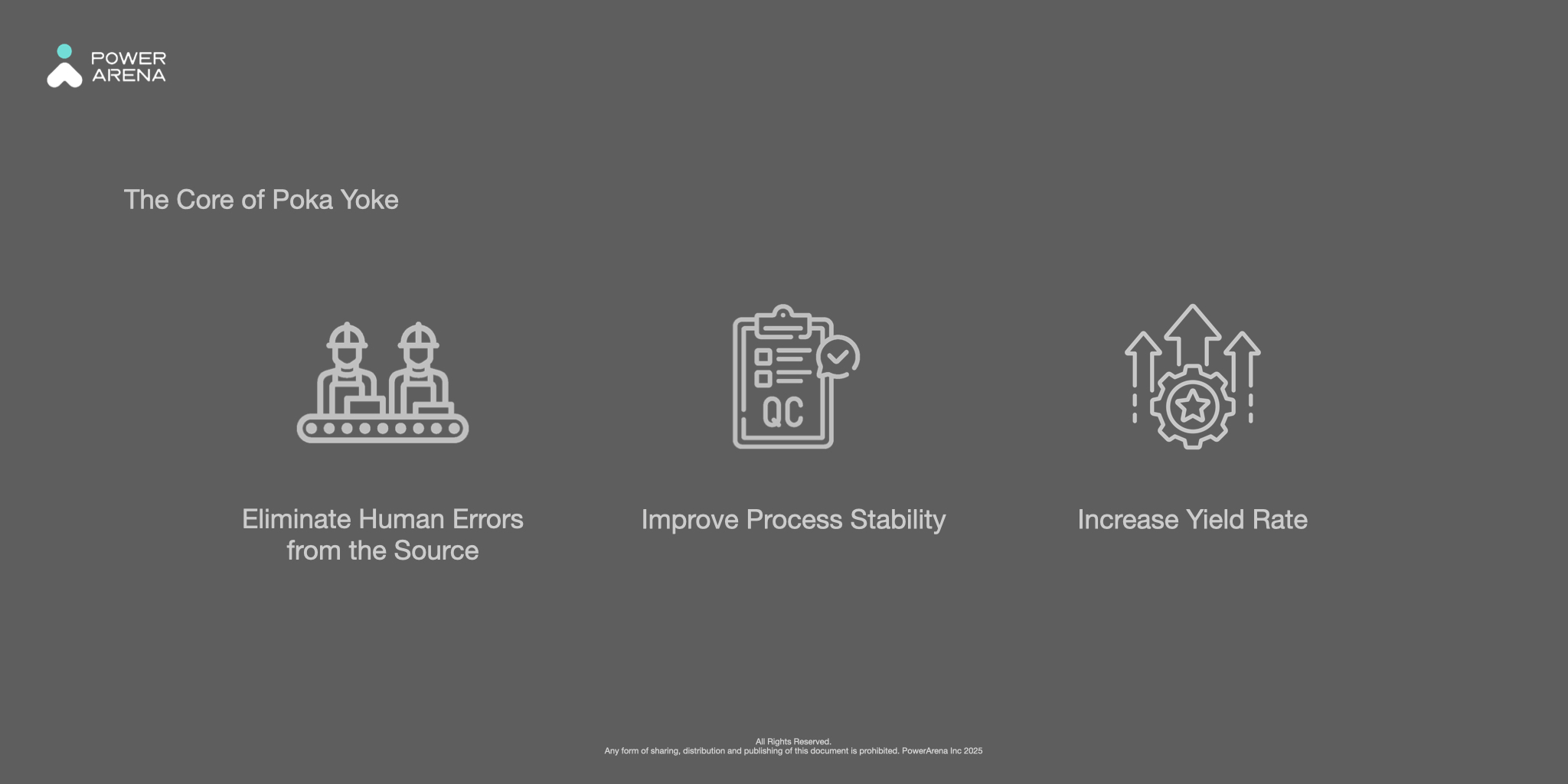
The Core of Poka Yoke
A motorcycle factory was facing recurring quality issues during final assembly—such as missed torque checks and incorrect part installation. A key issue occurred at the front fork workstation, where two operators worked in parallel. They had to apply oil and then tighten bolts, but once the bolts were fastened, it was impossible to confirm whether the oiling step had been completed. This led to hidden defects, such as rust developing over time.
To address this, the factory implemented AI automation with vision and motion tracking. The system automatically monitored operator actions in real time—detecting whether oiling was performed before fastening and verifying that all steps followed the SOP. If not, system will stop the process and send alert to the management immediately. It also provided visual traceability through recorded footage, giving managers full visibility and control over each task.
AI automation challenges
Digital infrastructure determines AI automation success
Digital infrastructure is not only crucial for ai automation deployment but also fundamental for companies’ digital transformation.
For AI to deliver value, factories must first establish the digital infrastructure needed to collect, store, transmit, and analyze data or other information, like video. The investments in equipment may include sensors on machines, camera for capturing process, network setup, and servers.
If a factory still operates largely with paper-based records, isolated machines, or untracked human workflows, AI will have nothing meaningful to analyze—leading to inaccurate outputs or system failure.
59% of high-level management with AI roadmaps stated that increasing IT infrastructure investments was a key element of their strategy.
—— Flexential 2024 AI Infrastructure Survey
Implementing AI requires comprehensive planning and execution across multiple levels of the organization. The key to success lies in management’s understanding of the current state of the business, setting transformation goals, and establishing long-term strategic plans.
AI Still Needs Human
Industrial AI today is far from fully autonomous. In real-world manufacturing environments, AI functions primarily as an advanced assistant rather than a complete replacement for human expertise.
In manufacturing, each factory has distinct products, processes, and management practices. Domain knowledge—often held by experienced operators—is key to managing unique workflows and edge cases. While AI excels at automation and quality control, it struggles with context and complex, unpredictable scenarios.
This means that, human oversight remains essential, particularly for reviewing outputs and making final decisions in non-standard or unexpected situations.
On-Site Resistance and Learning Curves
Adopting AI in factories often brings deeper management challenges. One key issue is aligning IT and OT teams to design solutions that truly solve real-world production problems—without slowing down the line or overcomplicating workflows. If a new tool is too difficult to use or doesn’t fit actual shop floor conditions, it risks becoming a burden rather than a benefit. On top of that, workers may fear job displacement or resist change, especially if the value of the technology isn’t clear. Even the most advanced AI systems need human trust and buy-in to succeed. That’s why training, communication, and usability are just as important as the tech itself when deploying AI on the factory floor.
The future of AI automation
Industrial AI for more precise automation needs
Among 1,800 manufacturing executives, 89% of companies plan to implement AI in their production networks. But only 16% of companies have achieved their AI-related targets.
—— BCG-WEF Project: AI-Powered Industrial Operations
AI in industry is rapidly moving away from generic, one-size-fits-all solutions toward highly specialized model tailored for specific sectors.
Rather than relying solely on large, general-purpose language models, companies are now prioritizing the integration of Gen AI and other advanced technologies directly into real-world industrial workflows. This transition is driven by the need for AI that addresses unique, industry-specific challenges—enabling more precise automation, targeted problem-solving, and measurable business value.
In this new trend, AI delivers real benefits only when it is customized to solve concrete problems within a given industry, transforming it from a theoretical tool into a core driver of competitiveness and efficiency.
Building Business and Factory Agility with AI automation
Download Report: 2025 Outlook - Singapore Reshapes SEA Manufacturing
In response to changes in geopolitical and economic environments, manufacturers are actively planning cross-border factory setups and supply chain restructuring. Vietnam, Thailand, and Malaysia are current targets, and regions like Saudi Arabia and Africa are also being considered as potential future destinations.
However, replicating the same production and management models across different locations presents a major challenge for businesses.
Read the Case Study: PowerArena’s Success Stories: Rapid Deployment Across Southeast Asia
ODM manufacturers note that, for notebook production, having a factory is not enough; factors such as a sufficient and suitable workforce, supply chain clusters, and logistics routes must also be considered.
── Digitimes, PC giants eyes Saudi Arabia for setting up plants as US tariffs supply disrupt
AI automation is a solution, significantly reducing reliance on labor in production and management, while overcoming language and cultural barriers. It standardizes processes and model production lines to be quickly replicated across different locations.
By integrating AI automation, businesses can effectively leverage data and ensure stable system operations, regardless of factory or country changes. This not only ensures stable and efficient operations during expansion but also enhances business resilience and international adaptability to face unforeseen challenges.
Table of Contents